AI-based Volunteer Engagement for Crowdsourcing Food Rescue Platforms (Predicting and Presenting Task Difficulty for Crowdsourcing Food Rescue Platforms)
Food rescue platforms rely on volunteers to deliver food to low-resource communities to fight food insecurity, but volunteer engagement is a main challenge in operations. This research looks to address the “right” demographic of volunteers to recruit, how to effectively engage them, and when to do so.
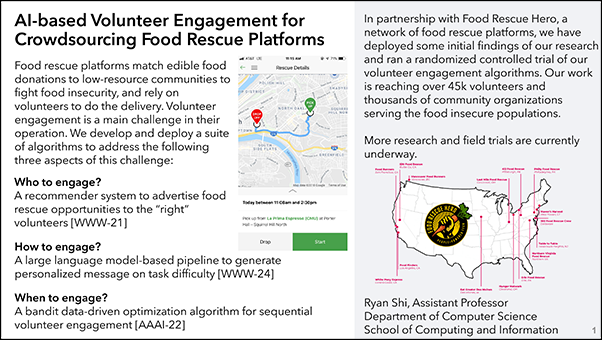
Research Methodology
A hybrid model with tabular and natural language data was used to predict the difficulty of a given food rescue trip. Focus group sessions with 10 volunteers and staff members were conducted where 6 different scaffolding methods present the ML model to users. Stakeholder interviews were also conducted to understand their perspectives on how to integrate such predictions into volunteers’ workflow.
Research Goals
This research aims to better understand how to improve volunteer engagement and retention through crowdsourcing volunteering platforms and predict how easy/hard a task will be for a volunteer.
Research Outcomes
Three LLM-based methods (natural language explanations, tag-based explanations, and augmented tag-based explanations) generated explanations for ML predictions. These results are currently being adopted with Food Rescue Hero to run a randomized controlled trial of the volunteer engagement algorithms.
Sponsors or Additional Collaborators
Food Rescue Hero
Carnegie Mellon University
University of Illinois